It’s likely that you’ve heard about digital twins as the global digital twin market size was valued at USD 7.48 billion in 2021 and is projected to grow at a compound annual growth rate (CAGR) of 39.1% from 2022 to 2030.
But, do you know all of the use cases? There is a lot of out-of-the-box thinking lately as it relates to the rise of the digital twin—so much so that we’re compelled to hop on this crowded content train to keep our audience tuned into the latest and greatest as it relates to this rapidly rising technology.
Originally, the concept of the digital twin started as the “Mirrored Spaces Model” which goes back to a University of Michigan presentation in 2002 for the formation of a product lifecycle management (PLM) center and subsequent university classes.
The phrase “digital twin” became more mainstream after being used in a roadmap report by NASA”s John Vicker in 2010, which focused on the idea of creating simulations of spacecraft and capsules for better testing.
Fast forward to today and digital twins are being used across all sizes of organizations and use cases to help companies understand complex products and model new ideas faster… and that’s just the tip of the iceberg.
What is a Digital Twin?
A digital twin is a digital representation of a real-world physical asset, process, or product.
It can be as simple (and as complicated) as that—and how they’re created and why vastly depend on the use case and where the company is at in the digital transformation journey.
Most of the time, digital twins are created by outfitting the physical real-world item with various sensors related to vital areas of functionality. These sensors produce data about different aspects of the physical object’s performance, such as size ratios, parts, and how those parts interact. They can also capture things like energy output, temperature, weather conditions, and whatever else is needed to design the best digital twin system for that particular item.
Once the data is collected, it’s analyzed and interpreted into a virtual model that can be used to analyze workflows, run simulations, study performance issues, and test possible improvements or new features, all with the goal of generating valuable insights in less time and with less capital.
Essentially, there are four separate technologies that comprise a digital twin:
- Internet of Things (IoT) sensors that gather data about the physical object
- Cloud infrastructure to store and process data gathered from the IoT sensors
- Artificial Intelligence and Machine Learning to analyze data, generate insights, and ultimately make predictions
- Extended Reality (XR) which encompasses augmented reality, virtual reality, and mixed reality
A digital twin is different from a simulation—while both utilize digital models to replicate processes and products, a digital twin goes much deeper and provides richer insights. It comes down to a matter of scale: a simulation usually shows one particular process in a specific period of time while a digital twin is meant to run a large number of predictive simulations in order to study different processes or functional changes or feature additions.
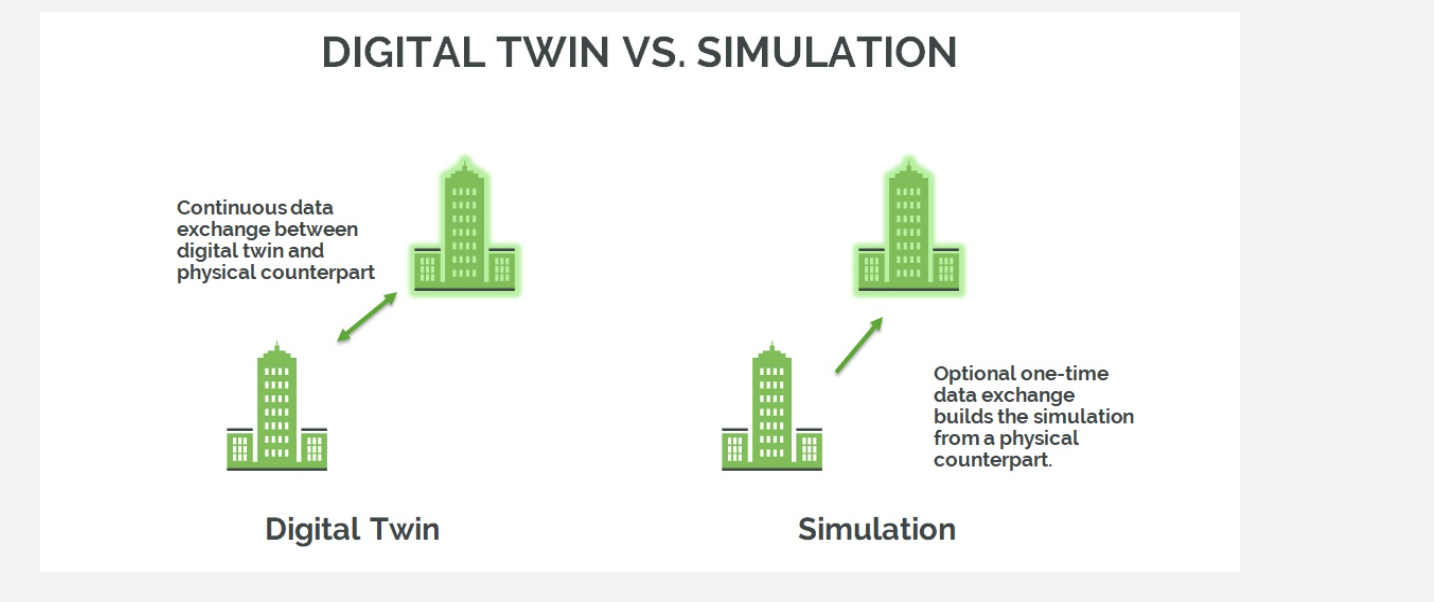
How are Digital Twins Used in Manufacturing and Why?
As it relates to manufacturing specifically, digital twins are an incredibly valuable technology as manufacturing plants have complicated pieces of machinery that constantly interact with each other. Beyond the physical machines, the overall processes of how products are made involve processes involving people, physical machines, and cloud-based technology.
Creating virtual representations of these highly complicated processes and machines enables manufacturers to find incremental improvement opportunities across the supply chain.
“A digital twin can help manufacturers understand the past, view present conditions, and prevent future problems, enabling informed decision-making through insight, analysis, simulation, and prediction.” - Dave Rhodes, VP of Digital Twins for Unity
Some use cases for digital twins in manufacturing include:
- Predictive Maintenance: Companies can estimate when a piece of equipment might fail so that corrective maintenance can be scheduled before it happens to decrease downtime.
- Decreasing Time to Market: By testing new features and even new products in a digital environment first, companies can test and learn faster.
- Increasing Efficiency: By modeling highly complex products and processes, companies can easier analyze opportunities for incremental improvement across the product lifecycle.
- Real-time Remote Monitoring: Companies don’t have to physically be near the machine to understand how it’s currently functioning.
- Encourage Innovation: R&D teams can explore new ideas and concepts and prototype inexpensively, which drives faster innovation.
But why are they used? What are the benefits of digital twins?
Whether you have a physical manufacturing plant or are a product company yourself, understanding the WHY is most important.
The benefits of using digital twins in manufacturing are typically focused on gaining a better understanding of products and customers.
That’s probably why Gartner predicts that ‘digital twins of a customer’ will transform customer experience—especially as we make our way into the newest wave of digital transformation with the Metaverse and web3 technology.
Risk and Challenges of Digital Twin Technology for Manufacturers
Because of the four separate technologies involved in creating digital twins, getting started with the technology comes with its risks and challenges—especially for manufacturers.
Companies who want to utilize digital twin technology must consider:
- Cybersecurity: Because all the tech that encompasses digital twins is connected to the cloud, companies must ensure they have the proper cybersecurity infrastructure in place.
- Data Quality: Before jumping into attaching sensors to machines, companies must understand what data they can collect and what the quality will be (including potential minor inaccuracy margins, etc.).
- Internal Training: Even if you choose to outsource setting up most of your digital twin technology, your internal team needs to deeply understand how this tech works, what success looks like for the project, and how to make sure the partner is providing best-in-class advice and services.
You don’t need to hire an expensive consultant whose niche is digital twins; there are great online resources that dive deeper into digital twin technology including:
- Mastering Digital Twins on Coursera (enrollment dates vary)
- Introduction to Digital Twins on LinkedIn Learning
- Digital Technologies and the Future of Manufacturing Specialization by the University of Michigan
- Digital Twin: A Comprehensive Overview (open enrollment/free)
These resources and other classes and certification programs can help prepare your internal team members for the process of implementing this Industry 4.0 technology.
Costs of Implementing Digital Twins
There’s a few different costs that relate to implementing and expanding digital twin technology with an organization including:
- IoT Sensors: These sensors stream data to cloud-based software for processing.
- Digital Twin Software: Digital twin software is the most direct upfront cost, as it’s what will display the digital twin model itself. There are many different technologies that have digital twin software solutions including Microsoft Azure, Apriori, and GE Digital.
- Software Integration: You don’t want to silo your digital twin data, meaning you have to invest in integrating your solutions, including your PLM platform.
- Training and Education: As mentioned in the previous section, companies must invest in training and education for those implementing new technology across the organization before, during, and after implementation.
The Five Levels of Digital Twin Deployment
Before we jump into how to get started implementing digital twin technology, we wanted to highlight the different levels of digital twin deployment to help inspire companies wherever they are in their digital twin (and Industry 4.0) journey.
The five levels or types of digital twins are:
- Descriptive Twin: This is purely a visual replica of the physical real-world asset.
- Informative Twin: The second stage starts to capture and aggregate defined data.
- Predictive Twin: Often using artificial intelligence and machine learning, this phase of digital twins uses operational data to gain future insights.
- Comprehensive Twin: This phase enables companies to begin generating ‘what-if’ future scenarios including new product development and additional features.
- Autonomous Twin: Begins to act on behalf of the users including making real-time decisions for companies.
How to Get Started with Implementing Digital Twin Technology
Every company has use cases for digital twin technology, and are all at different phases of their journey with not only digital twin technology, but with their shift to Industry 4.0 technology, practices, and policies holistically.
But, if you’re just getting your feet wet on this journey, this section is designed for you! There are definitely things you’ll want to consider before jumping into implementing digital twin technology at your organization.
As we said above, getting a deep internal understanding of how digital twins work is critical—because, without it, consultants and partners can lead you down a path that you may not be ready for, or is too big an undertaking for where you are in your journey.
We also suggest getting deep into how other companies have implemented this technology—both for the purpose of planning, but also to learn how to get started and the vast opportunities available to further explore once you get the basics down.
Once you have a firm grasp on all of the pieces of digital twin technology, here’s a general overview of how we’ve seen manufacturing companies get started:
- Determine the Purpose: Alongside your team, imagine the possibilities of a few small-scale projects you could pilot using digital twin technology that could potentially have the largest impact by using data-driven decision making. There are two main considerations in this phase: the potential ROI of the project and if there are major outstanding unclear processes that could potentially unlock value for customers or the company.
- Identify the Process: Next is to narrow down the pilot for the digital twin configuration that has both the highest possible value and has the best chance of being successful. Understanding internal bandwidth, external partners needed, and budget is critical at this stage.
- Define Success: Once you have the pilot project, you need to define what success looks like for this pilot. If not, you could keep extending the project implementation due to scope creep.
- Project Plan: Once you have your pilot and the success metrics, put together an agile project roadmap and plan that uses short sprints or cycles to keep the implementation process moving forward.
- Validate the Plan: Before you dive into the project plan, think about getting your roadmap externally validated. Many companies who create digital twin technology have experts on staff who can review your project plans and provide feedback and insights.
- Get Started: Now that you have a clear plan of action, it’s time to jump into the digital twin pilot and make sure your team understands why you’re doing this and the unique insights you hope to gain to get buy-in from all team members.
Once you’ve had success with your first pilot and analyze opportunities to iterate and improve, you can begin to operationalize and streamline digital twins in various areas of your company.
How to Take Your Digital Twin Technology Implementation to the Next Level
It’s easy to get ahead of ourselves when it comes to incredible new technologies—but when it comes to digital twins, it can be costly if it’s not a well-thought-out and planned process.
But, if you’ve already found success in your initial digital twin implementations and you’re ready to take it to the next level, we recommend following a few experts in the space including:
- Frank Pica, the CEO & Co-Founder of NativeAI who’s “disrupting the consumer goods industry using Digital Twins to fix a broken innovation system
- Dan Isaacs, CTO of the Digital Twin Consortium, a leading authority on digital twin technology
- Gurvinder Singh Ahluwalia, previous CTO for IBM North America, he has also worked at Motorola, Accenture, Sun, and Diamond Technology Partners (PwC) and is now the CEO of Digital Twin Labs
You can also follow the incredible work by these organizations:
- Digital Twin Consortium: They work with industry, academia, and governments to advance the overall development of digital twin technology.
- Digital Twin Forum: They host yearly conferences focused on digital twin technology.
Many say that the future of digital twin technology is feeding this IoT sensor data to all areas of the organization, enabling composable architecture. Composable architecture enables companies and users to adapt to changing business needs without waiting months for IT development.
Gartner predicts that by the year-end of 2025, 30% of available PLM applications will be built on top of composable technologies.
But traditional product lifecycle management platforms are no longer enough which is why best-in-class manufacturing companies are now shifting to product value management (PVM). PVM defines the distinction between a composable software platform and the lagging competitors who can’t make real-time decisions.
The world’s first product value management platform from Propel Software is built on top of Salesforce, which combines product and customer data in a single platform configured to meet your individual needs—including the data you’re gathering from your digital twin technology.
Keep reading about how composable architecture is predicted to be the next sea change in PLM software, putting certain product companies way ahead of the pack.